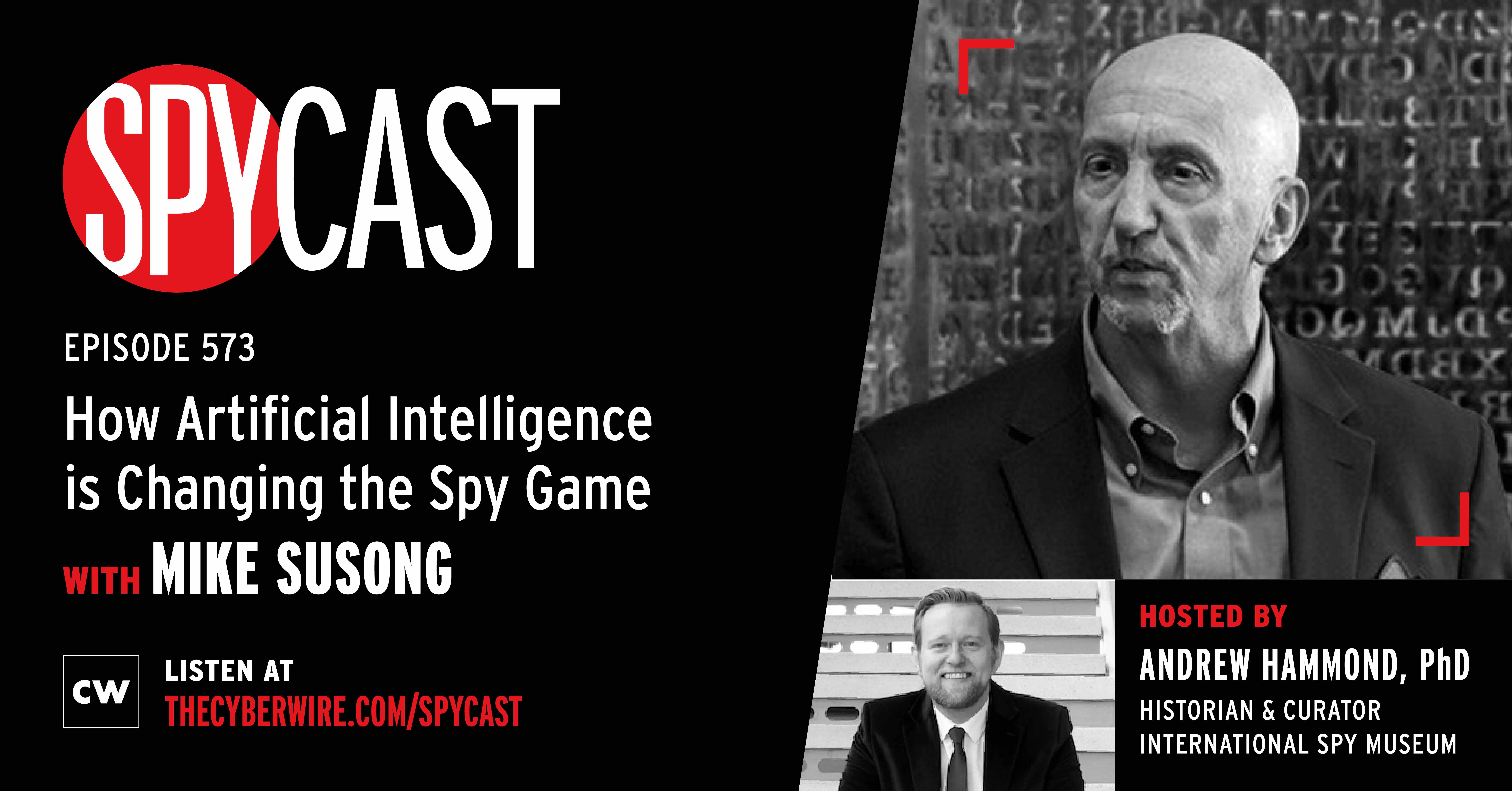
"How Artificial Intelligence is Changing the Spy Game" – with Mike Susong
Andrew Hammond: Welcome to "SpyCast," the original podcast on intelligence since 2006. I'm your host, Dr. Andrew Hammond, the museum's historian and curator. If you're looking for intelligence on intelligence, you've come to the right place. Every week, through engaging conversations, we explore some aspect of a vast ecosystem that looms beneath the surface of everyday life. Coming up next on "SpyCast"...
Mike Susong: My concern is - and it pains me a bit - is the intelligence community, which I love dearly and am proud of everything I did and proud of everyone who continues to serve, is it will take a lot of bureaucratic bravery and it will take a fundamental change, I think, in the way AI is applied to make it a revolution.
Andrew Hammond: Mike Susong as a former CIA operations officer - i.e., he recruited spies and stole secrets - who won the intelligence star for heroism in the field. Prior to that, he completed multiple combat tours with U.S. Special Forces. He left CIA to become an entrepreneur, pioneering cyberthreat intelligence and is currently a senior vice president for global intelligence with Crisis24. In the rest of the episode, Mike and I discuss what AI is and why it matters for intelligence, how AI can help and hinder intelligence officers in the field, the Ukraine-Russia conflict in AI, replicants, machines and robots and what you should and shouldn't be worried about.
Andrew Hammond: If you're new to the show, please subscribe to ensure you get your weekly high-level debrief. If you're already a member of the "SpyCast" community - and without you, the community, there is no "SpyCast" - please consider leaving us a five-star review. It'll only take a minute. And believe it or not, it really, really helps. The official podcast of the International Spy Museum, we are "SpyCast." Now, sit back, relax, and enjoy the show.
Andrew Hammond: Rather than getting into - straight into definitions and so forth, I think a good way to start off would just be give us an example of how AI is affecting the real world of espionage. So as a former case officer and someone who's now involved in the AI machine learning cyber field, how is it affecting the world of espionage? Do you have an example or a news story or a case or an event that you can share with our listeners?
Mike Susong: Sure, Andrew. And it really came up over the holidays and it - I think it was a great example. And it just so happened the wife and I were in New York City during the Christmas holidays. And we went to Radio City Music Hall, of course, to see the Rockettes. About a week later, the crowd queues up for Radio City Music Hall for each session. And there's hundreds of people, you know, flowing through multiple doors very quickly into the auditorium. And apparently, there was a lady who was with her Girl Scout troop. And she also happened to be an attorney with a firm that had a suit against the parent company that does Radio City Music Hall. And just from having been there and knowing the physical arrangement, you queue up on the street. Five seconds later, you're walking through the doorways, and there's a magnetometer and a metal detector a few feet in front of you. And very quickly, you go through the magnetometer, and you're in the music hall.
Mike Susong: When this lady goes through with her Girl Scout troop, literally within the 10 seconds it takes to turn into the building and go through the magnetometer, the security very politely intercepted her and explained to her that she was not allowed into Radio City Music Hall due to this pending lawsuit. It was all facial recognition. So, in other words, as hundreds of people are flowing into Radio City Music Hall off the street - and I would estimate in 10 seconds time - they were identifying an individual. And the lady said they knew her name. And they approached her by name and politely escorted her out of the facility. But I think as a case officer, that kind of sends a chill up your spine when you think about the ability to very quickly and accurately, with large volumes of people in a crowd, to be identified. So I think that was an excellent example of how AI used with the database of facial recognition can be a very potentially powerful defensive and offensive tool.
Andrew Hammond: So how would that work? So all the cameras would be feeding their information into a central database, and then artificial intelligence would be analyzing that for preset indicators of something? Or how does it all work? How does that shake out?
Mike Susong: Yeah, but take a step back. There's various sources for that information, you know, whether it's a driver's license image, whether it's other documentation - and really, when you think about it - and my numbers aren't current, but I'm confident that well over a couple dozen times a day, you're imaged - the ATM machine, going through the toll booths, walking in front of an office, going down the neighbor's street, and they have one of the camera doorbells on the front of their house. So being imaged and capturing those images and those images being available - again, social media, if you post an image of yourself. So there's a large number of sources that are available. Second step is then there's well-established formula to establish a facial geometry, which then, with the AI capability, can very quickly sort among, again, literally thousands, if not millions, of images and identify you with a - and it will then give a probability that you are who they think you are.
Andrew Hammond: I know you weren't involved in this personally, but just so I can try to get my head around it - so did that mean that AI was set up to look for the specific women, or was it - were there just the series of filters where if these types of people come up - pending law suits, known felons, whatever, then to refuse them access? Or how did the alarm bell go off, and how - what - how did they approach her?
Mike Susong: I would say this is very similar to the old profile book that the casinos in Vegas and other places kept, where they literally would identify who was a card counter or some other illegal. And you have to use that term very loosely in Las Vegas - some other illegal reason why you would not be allowed entrance into the facility. So they have in - just in turn identified people - in this case, a lady who legally represented her firm on the watch list. So it would be, as I say, as simple as that.
Andrew Hammond: So basically, as a case officer, this would be a countersurveillance nightmare when you're out there trying to stay undercover, trying to spot, assess, recruit and run assets. Then it's very difficult for you to make your way around a city or a country and do the work that you're meant to do if you're going to get flagged up repeatedly. So that must have - be very, very difficult.
Mike Susong: It absolutely complicates things. And I guess I would hearken back to, what is good tradecraft? Without AI facial recognition, you still seek to operate in plain view but in ways that don't alert the attention of anyone who's just casually observing. Of course, if you are under surveillance there, they're much more attuned to what your activities are. So it would just be that every country you're in is suddenly a hard target country as far as the aggressiveness of surveillance, just because of the pervasiveness of these tools.
Andrew Hammond: Let's take a step back for a second. And we are not computer scientists or data engineers or anything, but I do think it is helpful to give listeners that don't really know what AI is or have a loose conception of what it is to just give them something to hang their hats on. So what are we talking about when we talk about artificial intelligence?
Mike Susong: I'll start with artificial general intelligence, AGI, and that's basically the definition that a computer can handle any human intellectual task. It's memory, creative reasoning, abstraction, basically all the things that you attribute to the dystopian futures of HAL in "2001: A Space Odyssey" or Skynet with "The Terminator." There are no AGI systems out there - full stop. Now, I would be the last man to try to predict that future, but AGI does not exist. The next level down would be artificial narrow intelligence. And you'll hear it referred to as narrow or weak intelligence. And that's the ability of the algorithm to be able to perform a simple task with a high degree of integrity. Everything we're encountering today is narrow artificial intelligence - Siri, the selection that Netflix recommends for the next movie, robotics in factories and even self-driving cars. You know, it's - there's a hierarchy of, quote, unquote, "the self-driving part," but even that is considered narrow artificial intelligence.
Andrew Hammond: So at the moment, we're working within the narrow or weak artificial intelligence? That's correct?
Mike Susong: Yeah, that's correct. And if you go deeper - let's say we're in the narrow world - what people are now encountering - and maybe we'll talk later about ChatGPT - is basically machine learning using natural language processing. And natural language processing is the ability to communicate with the algorithm to query it in native language, whatever it is, English or otherwise. And so I think that's what - also is what's called a lot of the sensation with the public, rightly so, because the interface is much more friendly than trying to write a Python script or engage within the programming language.
Andrew Hammond: So tell me if I've got this right. So we've got human beings who can recognize patterns, learn from experience, draw conclusions, make predictions, do all of those things. And basically, artificial intelligence would be fully achieved when we have computers that can do all of those things that we can do just as well. And am I right in thinking that that moment would be called the singularity - when machines are smarter than humans?
Mike Susong: Yeah. If we go down the topic of singularity, that would be that state. And I think that's, again, a long way off, if achievable.
Andrew Hammond: OK. If achievable. So - and another thing they're trying to do, I think, is they're trying to get it to emulate and replicate human emotions and understand the - as I understand it, they have a very almost Spock-like way of communication. It's just - it's kind of cold. It's based on logic. It's based on reason. It's based on programming. But in the human world, there's lots of emotions and facial cues and all these types of things. So I guess one of the holy grails is to try to get machines that can deal with these types of human complexities, as well. Is that right?
Mike Susong: Yes. And kind of the essence of that is supervised learning and then reinforcement learning. And what that means is - to your point is emotions are so subjective, even though they're all - there are cultural patterns, if you will. And then to train the algorithm on seeing the micromovements in your face that are distinct to an emotion or at least can be attributed to a person's emotional state - and as with, if we're now talking about reading body languages, any expert will confirm that it's the holistic approach. It's not that I raised an eyebrow suddenly means I'm lying, or I grimace suddenly means, you know, something else. It's the whole - both the context, the circumstances you're in and then other actions that the individual takes. So when you think of that totality and then you say, OK, we're going to teach a machine to do that, you can see the complexity of the problem. But that said, these algorithms are becoming more and more powerful, and the processing power behind it is increasing also, as well.
Andrew Hammond: Wow. And bear with me here. So in "Blade Runner," replicants - and one of the issues there is that the replicants begin to experience emotions and question their own place in the world and so forth. Is the thought that, eventually, there will be machines that can get to that place where they start to think for themselves and potentially go beyond what we want them to do or become out of our control? Or is - I know this is, like, completely theoretical, but I'm just wondering what your take on it is.
Mike Susong: Well, "Blade Runner" is one of my favorite movies, but for all those reasons - again, we started off the conversation with AGI, artificial general intelligence. And I think that capability is so far in the future that it would be hard to predict that. And in that sense, I'm just not an authority to make speculations.
Andrew Hammond: Wow. And I read something. It was just a few days ago, in the news. Faces created by artificial intelligence are now more real than the genuine photographs, which is crazy. So something that's artificial is more real than the real. We're getting down all kinds of "Matrix"-like rabbit holes here. But it's crazy the way the ground is shifting underneath our feet, I think.
Mike Susong: You bring up a good point. And when you speak to faces and recognizing faces, you may be familiar with the concept of the uncanny divide or the uncanny delta. And that's the idea of when they are looking at robotics or what would be a physical interface between a humanlike robot, there's - if they're so close and you can still kind of in your mind say, this is not a human, everybody is OK with it. It's when they kind of get to that 95% but they still don't quite look human is when they're creepy. And so I think part of this effort on the creating the images that are more - look more human than human is an attempt to kind of bridge that divide so that the designers can know what is creepy and what's not.
Andrew Hammond: Just to bring it back to the intelligence community more specifically, help us understand the trajectory of AI in intelligence. So there's all these reports and summaries and briefings coming out just now, but when would this kind of thing pop up on the intelligence community's radar? So I believe the term artificial intelligence is invented in 1956, you know, and then it's obviously in very early, embryonic stages. But when does it pop up on the radar of the IC, or when - yeah, help us understand the evolution of the development of artificial intelligence out with the IC - so just more generally - and then the development of artificial intelligence within the I.C. and how that's mattered or not mattered more general development.
Mike Susong: OK. You bring up a good point on kind of how that's evolved. If you even go back to Alan Turing's initial paper back in 1950, which - what people refer to as the Turing Test and the movie, "The Imitation Game." At that point, obviously, still theoretical, but that was really one of the first discussions about what AI would be able to accomplish and how we would interact with the algorithm. That evolution really had a lot - it was kind of conflated with just computing power in the early - in the '50s and '60s. And obviously, computers into the '60s and '70s with mainframes were utilized extensively with the intelligence service.
Mike Susong: There was an AI winter when there were some kind of false starts on how algorithms would be structured, what path deep learning - and deep learning refers to the levels of the algorithm working, not that deep is better than some other form of the algorithm. And then really, kind of the last probably six years or so, we came out of the AI winter with both computing power - primarily with, like, systems like AWS where you can purchase computer power - and then some real breakthroughs on how neural networks work and how deep learning actually evolves and how you - I don't want to start down the rabbit hole, but back propagation, how you can then start to correct the errors that the algorithm produces. And so at that time, really at the same time, the intelligence community was an early adopter of these processes. And I would say, speaking from an outsider now, if you look at the intelligence disciplines, it would be - signals intelligence would be one of the early adopters.
Mike Susong: I mean, if you think about the problems and if you want to bucket them into signals intelligence and imagery intelligence and human intelligence, just the magnitude of the problem on the signals intelligence side, in some ways, dwarfs the others. You know, I just saw a statistic the other day that every day, there's 80 billion text messages. And so you multiply that by languages. You add in voice calls. You add in file transfers. You add in all the metadata that surrounds communication, and you can just see the magnitude of a problem that's perfectly suited for AI. And then with AI, it's not as simple as looking for keywords, but you then begin to be able to filter and monitor the vast amount of information that's flowing about for - or obviously for key indicators.
Andrew Hammond: The 1950 Alan Turing paper that Mike speaks of is called "Computing Machinery and Intelligence" and is surprisingly readable. It begins, (reading) I propose to consider the question, can machines think? From this, we get the famous Turing Test or Imitation Game, which aims to test the machine's ability to pass off as a human being. Many of you will have interacted with Turing's legacy probably in the last few days in the form of reCAPTCHA, that sometimes frustrating hoop you have to jump through online to prove you are not a robot. The CAPTCHA part stands for Completely Automated Public Turing test to tell Computers and Humans Apart. Alan Turing was an English mathematician whose work would prove influential in the future fields of computer science and artificial intelligence. He played a key role in the effort to break the Nazi Enigma code during WWII. Turing was arrested and punished for homosexuality in 1952 and tragically committed suicide two years later. He was posthumously pardoned. And in 2017, the British government enacted the Turing Law, which pardoned thousands more. He now features on the back of the British 50-pound note.
Andrew Hammond: Just to try to sketch out the broader development again - I'm sorry - I know that this is a lot of work, but I think just because it's so new for many of our listeners, it helps to dig into it a little bit more. So we have the Turing paper in 1950 and then the '56 conference at Dartmouth where they come up with the term artificial intelligence. And then there are other developments that go on. And then we go up to - I think this is a good example to maybe discuss. We came up to Deep Blue in '97. So Deep Blue is an IBM machine that basically beats the reigning world chess grandmaster, Garry Kasparov, in 1997, but Deep Blue is the weak or narrow artificial intelligence, isn't it? So could you just maybe discuss that example to help us get our heads around artificial intelligence more generally? And what would be the - thinking about Deep Blue, people were thinking, wow, this is something we never thought would happen, a chess grandmaster being defeated by a machine. So yeah, just help us kind of understand Deep Blue and the Rubicon that was crossed when it beat Garry Kasparov.
Mike Susong: And I think that does give a good illustration about the application. But again, it's still narrow. It was a chess board. And I'm probably the world's worst chess player, but you can conceive that there's only a finite number of moves that can be applied. And what little I know about grandmasters is their ability to use different strategies and different techniques that have been used before is what then gives them the edge and that they play at that level. So again, we're going back to the reinforced learning model. Deep Blue was basically trained to learn every permutation. And when Kasparov did a certain move, it could anticipate, OK, this is one of three variables that - what will be the next five moves? As many grandmasters do, they're thinking several moves ahead. And so I think this was the application that really brought it to the public's attention. Similar models have been used on go, you know, which is an Asian board game, which is - arguably has more permutations and more nuance than chess. And I think it is - I think the grandmaster was Korean and that the algorithm beat him, as well.
Mike Susong: So these - I think it brings to the public eye the capabilities. But again, remember, if you think about a chess board or a go board, that's a very finite universe. Maybe more than we can comprehend, but it's still a finite universe. And so its application is still narrow. On the other hand, without dismissing the definition of narrow, if you have 20 algorithms and each one of them are narrow, suddenly, they don't look so narrow if each one of them has a particular task and a particular skill that they accomplish.
Andrew Hammond: And let's go on to ChatGPT, which you mentioned earlier, which is all the rage in the heat of the moment. And in the research for this episode, I actually signed up. And I put in, write - I was just being playful - write a sonnet - you know, a 14-line poem on espionage. And it'd done such a good job, I posted it on social media, on Twitter and on LinkedIn. My hopes weren't particularly high, but it was so good. That's the type of thing that it could take human beings years to get that level of fluency writing poems or - and doing all the things that you need to do, but ChatGPT just spat it out in five seconds or so. I'm just wondering, have you used it? Can you tell the listeners what it is? And what implications does it have for the intelligence community?
Mike Susong: Sure, Andrew. And I read your poem. You and...
Andrew Hammond: Oh, you did? OK.
Mike Susong: ...ChatGPT are quite eloquent.
Andrew Hammond: Well, ChatGPT's poem had nothing to do with me (laughter).
Mike Susong: Well, you designed the prompt. And that's a whole 'nother conversation about intellectual property, as to who owns it. You designed the prompt. No, yeah. ChatGPT, it's certainly the rage. And again, the value of it is it's exposed the broader public to a friendly user interface for a narrow AI capability. But ChatGPT is chat generative pre-trained transformer. And that's just the model that was used for what people are using today. The original GPT 3.0 was - I think it was probably 2000 when it was released. And so this is actually 3.5, and there'll be a GPT 4 probably later this year. And there's nothing ominous about a .4 as much as it'll just to - have more capabilities. So some of the problems people are encountering on syntax or if you're using Stable Diffusion on some of the imagery, it's - it'll be a little more defined. So - but certainly, ChatGPT is a good example. And people are using - I would say in a phrase that it's more heat than light.
Mike Susong: It is, again, good at narrowly defined task, maybe, being generous, equated to a smart intern if you were an intelligence analyst. They still need close supervision, broader education and reinforcement on what and how they're writing. But if we want to kind of apply this to the intelligence process, certainly, my company has embraced it fully on using AI to monitor the world and to then begin to aggregate the key indicators that then a human analyst can best put the pieces together and make the detail analysis. But again, as we use the example earlier with a signals intelligence about the vast amount of data and the speed with which it's generated, humans can nowhere approach the ability to manage that. I'll quote the title of a '60s poem, "All Watched Over by Machines of Loving Grace." The idea that the algorithm is doing that first cut according to your criteria, and then it can deliver then to the human analyst a rough approximation. And when you look at ChatGPT, that's really what we get in most cases. And then the application of the subject matter expert and other criteria - you know, our collection requirements, the particular question that the decision maker asked that will then help refine the final product.
Andrew Hammond: Wow. And just briefly there, can you tell us what stable diffusion is?
Mike Susong: Sure. Stable diffusion is a similar prompt, but it generates images. DALL-E - D-A-L-L dash E - kind of a play on words with "Wall-E," the movie, and Dali, the artist, does similar. You type in a prompt - Mickey Mouse, you know, ascending the Himalayas in an impressionist style - and it will generate usually three or four images as its best estimate. So it's similar to your poem, but for artwork. So now, Andrew, you can start your own art gallery.
Andrew Hammond: That actually sounds - I really want to see that now, Mickey Mouse ascending the Himalayas in an impressionist style. I think that might be what I'm going to do after this episode. And just briefly, before we move on, one other thing that you mentioned that I meant to just pick up on was neural networks. Can you just tell our listeners what neural networks are?
Mike Susong: Yeah. And again, the data scientists are usually quite disciplined about the terminology. And it's the layman, such as I, and the public that usually conflate them. And a short version - let me take a step back to make this as simple as I can. Let's say you're trying to teach the algorithm to see a cat - to identify a cat. Each neural network, each neural node would be as - again, this will be oversimplified. But each neural node would be two eyes, four legs, certain height, fuzziness. And you can go into all the nuance that you want. But then each network and each neural node can only then, in an almost binary way - you can be more complex, but in an almost binary way, two eyes, not two eyes, four legs, not four legs. And so you can just have millions of dimensions. And then the conclusion is cat, not cat. That's, you know, in a very crude manner, what a neural network is. It's just a way of categorizing the task that you're trying to teach the algorithm on.
Andrew Hammond: I think it would actually be quite interesting, if you're amenable to it, to tell us a little bit about your company and how it uses artificial intelligence.
Mike Susong: Sure, and I'll appreciate the opportunity to do so. I'm the senior vice president for global intelligence for Crisis24. It's a GardaWorld company, and we're globally located. Our company provides geopolitical intelligence analysis to 700-plus global organizations - everything from NGOs to large corporations. So we're a completely open-source, proprietary intelligence company. And we use a combination of human intelligence and artificial intelligence to produce our products for our clients on a 24-hour basis. As we've discussed earlier, just the scale of information, the speed of information as it's available, has surpassed what a human analyst can do. So we've applied, with our data science and artificial intelligence team, that capability to our mission.
Mike Susong: One point I'll make - and I think it becomes clear when people play with ChatGPT - the quality of the information that it's trained on is the quality of information that you get, you know? Even decades ago, when I was programming, it was garbage in, garbage out. So our company has the luxury of having 25-plus years of highly curated human intelligence. It's a glorious training set I would play. So our ability to train the algorithm on - by country, by event, by security category is really quite remarkable. And we've seen leaps and bounds in the result of that from our analysis.
Andrew Hammond: It would be fun to walk through, maybe step by step, ways in which AI affecting your former job as a case officer. I know there's only so much that you can talk about, but help us understand it. So things like your identity, your cover or legend, how you would spot, assess, recruit and run assets - could AI help you do that? Could it help you spot lies? Could it help you with covert communications? Help us understand, stage by stage, how you could see AI potentially affecting work as a case officer. And it's fine if there's no way that AI could do this. But if there is, it would be interesting to know. And maybe discuss the tradeoffs - like, you could use it for this theoretically, but I don't think it ever will be because blah, blah, blah.
Mike Susong: You know, it's an excellent question and one I think frequently about. As a case officer, you know, is - for the audience - the job of running assets, recruiting spies and stealing secrets. The application of AI is having a profound effect and will continue to do so. And in no particular order, we mentioned earlier about facial recognition being used to identify an individual. If you're in disguise or if you're just hoping not to be identified, that pervasive surveillance limits your - potentially limits your operational capability when you need to go operational. If you look at cover and legend - the identity that you're assuming in order to operate in a certain place - there's two sides to that coin. If you are operating undercover, you should have a social media presence, and you should have a digital - you should have digital exhaust - you know, things about websites you've gone to or purchase records on Amazon. If you don't, then that can very quickly erode your cover. Let's say I'm - I claim to be John Smith from whatever country and in whatever profession. There should be some footprint of that in the digital world. And if there's not, then that - again, it arouses suspicion of the local services. So then and there - and, again, all of this would be managed by artificial intelligence. It's not somebody trying to read through Facebook pages to identify someone.
Mike Susong: When you look at spotting, assessing and recruiting, it would be similar. We've all, jokingly, probably exclaimed on - we're amazed what people put on social media about themselves and others. And, again, as one dimension, that's a way to spot and assess or look for vulnerabilities or look for access that an individual may have that we're seeking out. So again, you apply the AI algorithm to helping you spot for and watch for these type of capabilities - is another tool. And, again, it multiplies the ability to look for a potential asset. For polygraphy - lie detectors - I think that dimension there is just the tools that AI would enable the polygrapher to be able to be a - have a more refined assessment of deception detected or not detected. When you think of the biometrics that are being observed - again, you could add, again, facial micromovement. You could add body language. You could add a lot more cues that just even the best polygrapher can't observe simultaneously. So again, if you think from an intelligence point of view, the AI capability is, again, more sets of brains and eyes and ears.
Andrew Hammond: In-Q-Tel was founded in 2016 as a venture-capital firm that would invest in high-tech companies, thereby allowing the CIA and the U.S. government to benefit from cutting-edge developments that enhance national security - or, as they put it, combining the security savvy of government and the can-do curiosity of Silicon Valley. AI and machine learning are a huge part of this, along with data analytics, autonomous systems, and what is called the fourth industrial revolution. They are headquartered in Arlington, Va., and people like former CIA director George Tenet and former chairman of the joint chiefs of staff, Mike Mullen, are on their board. Fun fact - the Q in In-Q-Tel is a direct play on the gadget man from the "James Bond" series, Q. Links and resources for Alan Turing and InQTel can be found at thecyberwire.com/podcasts/spycast.
Andrew Hammond: Is artificial intelligence a revolution that's happening to the intelligence community? How would you categorize it? It's going to rip up the playbook and so forth, or all the way to the other end of the spectrum, which is it's not really going to change that much. And obviously, neither of them are probably true. But where would you land on the spectrum?
Mike Susong: For technology in general, I think there's always an evolution. If we look at agrarian societies going to industrial, going to computer, there's an evolution. But there are seminal moments even in those, where you have to say, OK, that was the moment things changed. And I wouldn't say it's always - you're a professional historian, so you know this better than I. It's hard to read the label from the inside of the bottle. When you're still in the middle of something, it's hard to see the change that's taking place. But I do think it is fundamental.
Mike Susong: Now, I'll have to get on my soapbox for a moment. My concern is - and it pains me a bit - is that the intelligence community, which I love dearly and am proud of everything I did and proud of everyone who continues to serve - is it will take a lot of bureaucratic bravery, and it will take a fundamental change, I think, in the way AI is applied to make it a revolution. I think it'll be - it runs the risk of being incremental or even window-dressing changes versus really, fundamentally advancing our ability to defend our national interests using AI as a tool.
Andrew Hammond: At the moment, generally speaking, it's more of an add-and-stir approach. Let's try to fit it within the structures and the processes and the culture that's already there. I'm speculating here, but I'm just wondering how you see that change coming, if at all.
Mike Susong: I think the change can come. And, as I say, I'm absolutely committed to the intelligence community. From the outside, as a public partner, I - my strategy is, frankly, to use the war in Ukraine as the hammer because when you look at open-source intelligence and what is being accomplished there, as far as by citizen analysts, I think it is unremarkable. Sadly, we have a living laboratory in Eastern Europe. But if you don't mind, I'll just give some examples of how that is, I think - could be a wake-up call for the IC on how to apply open-source intelligence - use that as the wedge for some bigger changes.
Mike Susong: But if you look at just in the early days of the war, the military intelligence analyst would be looking for order of battle and where forces are massing, and you would use overhead systems or maybe a human intelligence analyst or imagery intelligence. Google Maps was reporting the traffic jam at 3 o'clock in the morning on the 23 of February across the border in Russia from Ukraine. And previously, the day or two before, social media individuals had taken photographs of infantry fighting vehicles and armored personnel carriers in that same location. So there you have it. Google Maps tells us where they're crossing the border. In concert with that, you could look at where the traffic jams continued and go to some of the commercial satellite companies. And for a couple hundred bucks, I'm suddenly the National Geospatial Agency. I have imagery resolution down to 30 centimeters, which is about a foot, and the evidence is in front of me. I can make that assessment versus a national overhead system.
Mike Susong: You look at a signals intelligence mission of where units are inside Ukraine. Russian 19-year-old men - being what any 19-year-old man is - they were all on VK and Telegram and Instagram and Tinder, trying to pick up Ukrainian girls, and, whoops, they forgot to turn off geotagging. And so a lot of Russian units were identified quite clearly and accurately by this to social media. There's locations where it's a, quote-unquote, "abandoned" airfield or facility. And the soldiers have been jogging wearing their FitBit, and they've basically drawn a box around the building or airfield or line of armor that's parked in the motor pool.
Mike Susong: Again, all of this is open-source intelligence. And, you know, my favorite - somewhat lethal, but favorite example is a young 15-year-old boy in Ukraine who, when the Russian armored columns were making - advanced on Kyiv, him and his father, they went out and he flew his inexpensive drone up over the battle lines, added the lat-longs using a commercial mapping program and sent the coordinates to the Ukrainian artillery. And they literally decimated a good section of a Russian armored column - a kid using a $300 drone. And, as I joked, it's a good thing it wasn't a school night, or the Russians might have taken Kyiv if it hadn't been for him and his drone.
Mike Susong: So when you look at all those capabilities - and I'm not implying the IC needs to start buying $300 drones, but we've just talked about every one of the INTs using commercially available information and open-source resources to accomplish that. The IC has to get their heads around working with OSINT in a constructive way. You hear arguments about - we need to make another discipline, another center. Let's form a committee. And, to me, that's all motion to give the illusion of progress, and that it's just - needs to be embraced and acknowledged that what is classified should be the minority, closely held and protected, but then the vast majority of information can be achieved through open-source partnerships with private companies or just that capability within the IC.
Andrew Hammond: One of the reports that I mentioned earlier - so talks about entering an AI era. And in that, it says that the problem is not the technology. The problem is the culture. So this is a report that's informed by lots of seniors, farmers and so forth. Don't know - as someone that used to be a member of the tribe, why would culture - not technology - be the problem?
Mike Susong: I think we touched on it a moment ago when we talked about the unnatural nature of open information. And there's a bias. It probably is endemic. There's a bias towards the classified information, like it has more value or merit. I will absolutely agree that there are times, strategically, that that piece of classified information is absolutely the gem that lets a decision-maker make a decisive and bold decision. But more times than not, it's just a matter of the best vetted information, regardless of where it came from. So I think it is culture. If your work day is - I'm a U.S. citizen. I have a security clearance. I'm working inside a SCIF - a secured, compartmentalized information facility. I'm on a classified network, seeing, by and large, only classified information, which is a TS code word and a final report. All those things can be an impediment to changing your culture. That's the routine. That's what I know. That's what is - the way things are done and have been done for decades, to say, well, I could have just sat outside in an office somewhere in the D.C. area and done the same, you know?
Mike Susong: A good example is my - a good example is our intelligence team are over 75% international citizens. The minority are American citizens. I have no doubt as far as the integrity and reliability and intelligence of this intelligence team that we have, but not a one of them would get a security clearance. And that has nothing to do with their character, it's just - it wouldn't happen. So if I can take that team - 100 plus - commercial imagery, an AI capability to watch social media, watch academic papers being written in Amsterdam on Boko Haram in Dutch presented at a forum in Paris, and incorporate that into my analysis, we're coming real darn close to being able to match national systems, I would argue.
Andrew Hammond: I think one thing that I wanted to ask was, whenever you do research on AI or you look at AI, one of the things that always comes up is - are we all going to lose our job? Is there going to be a time where human beings or a large percentage of human beings are going to be redundant? They're not going to be needed anymore because AI is going to come along and do a lot of things, but there's not necessarily going to be replacement jobs because AI will be able to do so much more than we can do and do it better and quicker and with less risk to us. What's your take in that? I mean, either more generally or with regards to the ICs, does the IC need to be slimmer and leaner in the era of AI, or is that not really the right question to ask?
Mike Susong: No, I think it's a very valid question to ask, and it's certainly on people's mind. And I'll go down both those paths in general and with the IC. In general, I'm an optimist. When we looked at the agrarian society going to an industrial society, agricultural production increased. You need fewer farmers. But then there became jobs in factories, and then factories gave way to white-collar jobs. And blue-collar jobs became more automated and had higher technical requirements, and so those jobs evolved. And I think it's the same way with AI. Things that can be repetitive, that encapsulate a great deal of information to make a decision that, frankly, the human mind is not best designed for are tasks that AI will take over, and then we will just move up the stack. And I think that applies with the IC. And I keep harkening back to the intelligence team that we have.
Mike Susong: But that's the whole objective - is I don't want somebody with a Ph.D. in Asian studies having to monitor for indications of a port strike in Vietnam. I want them to be thinking about the trends of industrialization in the Asian Tigers and how that will portend great competition in the theater with the Chinese. That's still a very human task and something that you need the human's capability to address. So can you find economies in the IC? I would think so. But again, hopefully the idea would be then to apply those humans to more sophisticated tasks.
Andrew Hammond: Help us understand the AI landscape with regards to intelligence. So we spoke a lot about American intelligence. How does AI play out across the rest of the world? So it seems like China is going for AI in a big way. The United States is going for it in a big way. Is AI going to create another level of technological differentiation between great powers and the rest? Is AI going to be something for the superpowers and the rich? Are there are a lot of barriers to entry? Is it something that - it doesn't matter if you're very low in the Human Development Index - you can still use AI and, you know, it's not let America do the research, and then, when it filters out, we'll take advantage of it? Help us just understand the effect not just on American intelligence, but across the whole intelligence ecosystem.
Mike Susong: That's a very good question. And I would - I'll use the analogy that I saw in Africa. You would go down the street, and there would be, literally, plain, old telephone service wires strung across the trees and across the tops of buildings. And then you go out into a village somewhere, and they have cell service. So I think what we'll see to some degree with AI, similarly, is technological leaps. As you implied, America and others will do a lot of the heavy lifting, build out a lot of capability that can then be commercialized and economized. And I think you'll selectively see other intelligence services pick up those pieces and capabilities. So the cautionary tale for a case officer is don't assume because you're in country X and they're on rolling blackouts, don't think that they don't have facial recognition at the airport, or they don't have imagery surveillance capability over the apartment building where you're trying to establish a safe house. So I think, obviously, the preponderance will be in countries that can resource and deploy those capabilities. But I would not for a moment assume that any operational environment that you will go into can very quickly change over the next 12 to 18 months.
Andrew Hammond: OK. Wow. I think the last thing I wanted to ask you, just as we sign off - I read that something like 20,000 devices work with Alexa. So what is Alexa? Is Alexa AI? And how worried should we be about its control over these devices?
Mike Susong: That's a good question. I would say there probably are that many apps that can work with Alexa - everything from your Fitbit to the lights to the home alarm system. Alexa's narrow AI. You can easily stump her with a question or a request, but you really touch on something I think is another dimension of the impact on espionage. And when you look at the Internet of Things, the Internet of Things is basically anything, other than a computer or a smartphone, that emanates data. Again, that could be the open and closed switch on your door. That could be your Fitbit, whatever that may be. And so all of that is now being meshed into the larger body of knowledge that's available out there. So one, just - that's just more things to be aware of, and it's a potential risk. You know, let's look at it from an operational point of view. If I needed to do, you know, what's euphemistically called a black-bag job on a building and I know that the guards are - happen to wear Fitbits and if I can access that, I'll just wait till he goes into REM sleep, which is the deepest sleep. And that would be the most likely time for me to enter the building without him awaking and being aware of what's going on. And so all those things that are emanating information or that we're communicating with potentially hold a risk for espionage or can be a benefit for espionage.
Andrew Hammond: Well, thank you so much for helping us break all of this down. It's been a real pleasure, as ever, to speak to you.
Andrew Hammond: Thanks for listening to this episode of "SpyCast." Please follow us on Apple, Spotify or wherever you get your podcasts. Coming up in next week's show...
Alan Kohler: Oh, yeah. Yeah. That unit - that exact unit exists. It's a parallel unit to the criminal serial killer unit, and we use them extensively. So, for example, when I was a supervisor in New York, we had the Ghost Stories cases, the Russian illegals cases up there. We brought the behavioral analysis folks up to New York. We've been looking at these subjects for years. Now we're finally going to get to get in front of them and talk to them. How should we do this? Can we approach it this way? Should we use this wording? There's a lot of psychology that goes into it, and that helps me, as a supervisor, pick the person that's going to go in the room.
Andrew Hammond: Next week's guest is Alan Kohler, FBI assistant director for counterintelligence, which means he takes the lead in all counterintelligence investigations across the U.S. government. If you enjoy the show, please tell your friends and loved ones. If you have feedback, you can reach us by email at spycast@spymuseum.org or on Twitter at @INTLSpyCast. If you go to our page, thecyberwire.com/podcasts/spycast, you can find links to further resources, detailed show notes and full transcripts. I'm your host, Andrew Hammond, and you can connect with me on LinkedIn or follow me on Twitter at @spyhistorian. My podcast content partner is Erin Dietrich, and you can follow her on Twitter at @erinpubhist. The rest of the team involved in the show is Mike Mincey, Memphis Vaughn III, Jo Zhu, Emily Coletta, Afua Anokwa, Elliott Peltzman, Tre Hester and Jen Eiben. This show is brought to you from the home of the world's preeminent collection of intelligence and espionage-related artifacts, the International Spy Museum.